The Ultimate Guide to Image Labeling: Enhance Your Data Annotation Process
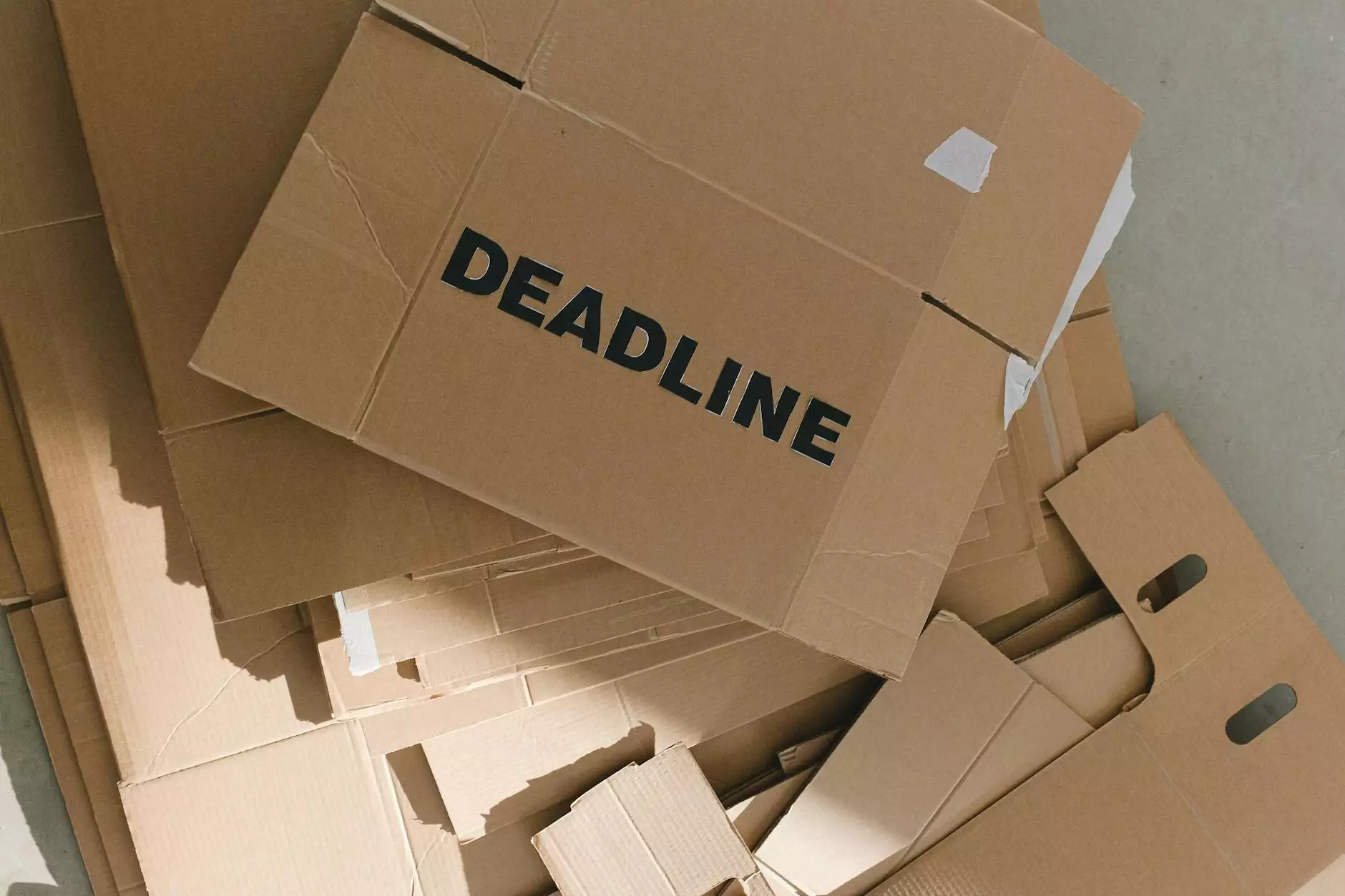
In today's data-driven landscape, the importance of effective image labeling cannot be overstated. As businesses across various sectors increasingly leverage machine learning and artificial intelligence, the accuracy and efficiency of their data annotation processes become critical. This comprehensive guide will explore the intricacies of image labeling, its significance, and how you can optimize your efforts with advanced data annotation tools and platforms, such as those offered by KeyLabs AI.
Understanding Image Labeling
Image labeling is the process of assigning meaningful labels or tags to images. This practice is essential for training machine learning models, particularly in the realms of computer vision and artificial intelligence. Well-labeled images enable algorithms to recognize patterns and make decisions based on visual data.
The Necessity of Image Labeling in Machine Learning
Machine learning models require vast amounts of data to learn from, and image labeling ensures that this data is correctly categorized. Without accurate labels, the model's ability to learn or make predictions is severely compromised. Here’s why image labeling matters:
- Improved Model Accuracy: Labeled images provide clear guidance for models, leading to higher precision during training.
- Better Performance: Models trained on well-labeled datasets perform better in real-world applications.
- Application Versatility: Image labeling is critical for various applications, including facial recognition, autonomous driving, and medical imaging.
Key Techniques in Image Labeling
There are several techniques employed in the image labeling process, each suited for different types of projects and goals. Below, we’ll highlight the most commonly used techniques.
1. Bounding Box Annotation
This technique involves drawing rectangular boxes around objects within an image. Bounding boxes are typically used in object detection tasks, allowing algorithms to learn where objects are located in photographs.
2. Semantic Segmentation
Semantic segmentation goes a step further by classifying each pixel in the image. This method provides more detailed information about the objects within the image and is particularly useful in applications such as medical imaging or scene understanding.
3. Instance Segmentation
Instance segmentation identifies and delineates each object instance in an image. Unlike semantic segmentation, which classifies pixels into categories, instance segmentation can differentiate between multiple objects of the same class.
4. Keypoint Annotation
This involves marking specific points of interest within an image, such as joint locations in human poses or control points in facial recognition. Keypoint annotation is essential for applications requiring precision in object tracking.
Challenges in Image Labeling
Despite its importance, image labeling presents several challenges:
- Time-Consuming: Manual labeling can be a tedious process, especially with large datasets.
- Subjectivity: Different annotators may interpret labels differently, leading to inconsistency in the dataset.
- Scalability: As data grows, so does the need for efficient labeling methods that can keep pace.
Best Practices for Effective Image Labeling
To overcome the challenges associated with image labeling, businesses must adopt best practices that enhance the quality and efficiency of the process:
1. Utilize Advanced Annotation Tools
Invest in a robust data annotation platform like KeyLabs AI that offers machine learning-assisted labeling. These tools can significantly reduce the time and effort required for data annotation while increasing accuracy.
2. Implement Quality Control Measures
Establishing a quality assurance protocol is essential to maintain the integrity of your labeled data. Regular audits and feedback loops can help mitigate errors and ensure consistency across annotations.
3. Training and Guidelines for Annotators
Providing thorough training and clear guidelines to annotators is crucial. Well-defined labeling criteria help annotators make informed decisions, reducing subjectivity.
4. Leverage Automation Where Possible
Incorporate automated labeling solutions to handle repetitive tasks. Augmenting manual labeling with automation can accelerate data processing times and improve throughput.
Benefits of Streamlined Image Labeling
Implementing a refined image labeling process can lead to numerous benefits for your organization:
- Increased Productivity: Efficient labeling workflows enhance productivity, enabling your team to focus on core tasks.
- Cost Savings: By streamlining the labeling process, businesses can reduce labor costs linked to data annotation.
- Faster Time-to-Market: High-quality labeled data allows for quicker model training, leading to faster deployment of AI solutions.
Case Studies: Successful Implementation of Image Labeling
Many businesses have realized significant advancements through effective image labeling. Here are a couple of notable examples:
Case Study 1: Autonomous Vehicles
Image labeling in the automotive industry, particularly for self-driving cars, entails annotating roadway signs, pedestrians, and other vehicles. Companies leveraging advanced data annotation platforms have successfully improved their autonomous vehicle systems, achieving higher accuracy in object detection and improved safety measures.
Case Study 2: Healthcare Imaging
In healthcare, precise image labeling facilitates the development of diagnostic tools. By annotating medical images with conditions such as tumors or fractures, machine learning models can better assist radiologists in identifying and diagnosing various ailments, ultimately enhancing patient outcomes.
Final Thoughts
As the demand for machine learning and artificial intelligence capabilities continues to rise, the role of image labeling will remain pivotal. Companies must prioritize effective data annotation practices to stay competitive in their respective markets. By harnessing advanced data annotation tools and adhering to best practices, organizations can optimize their image labeling processes, leading to improved model performance and more informed decision-making.
Explore how KeyLabs AI can assist you with image labeling and other data annotation needs, providing you with the tools necessary to thrive in this data-centric world.