Harnessing Malware Machine Learning for Enhanced IT Services and Security
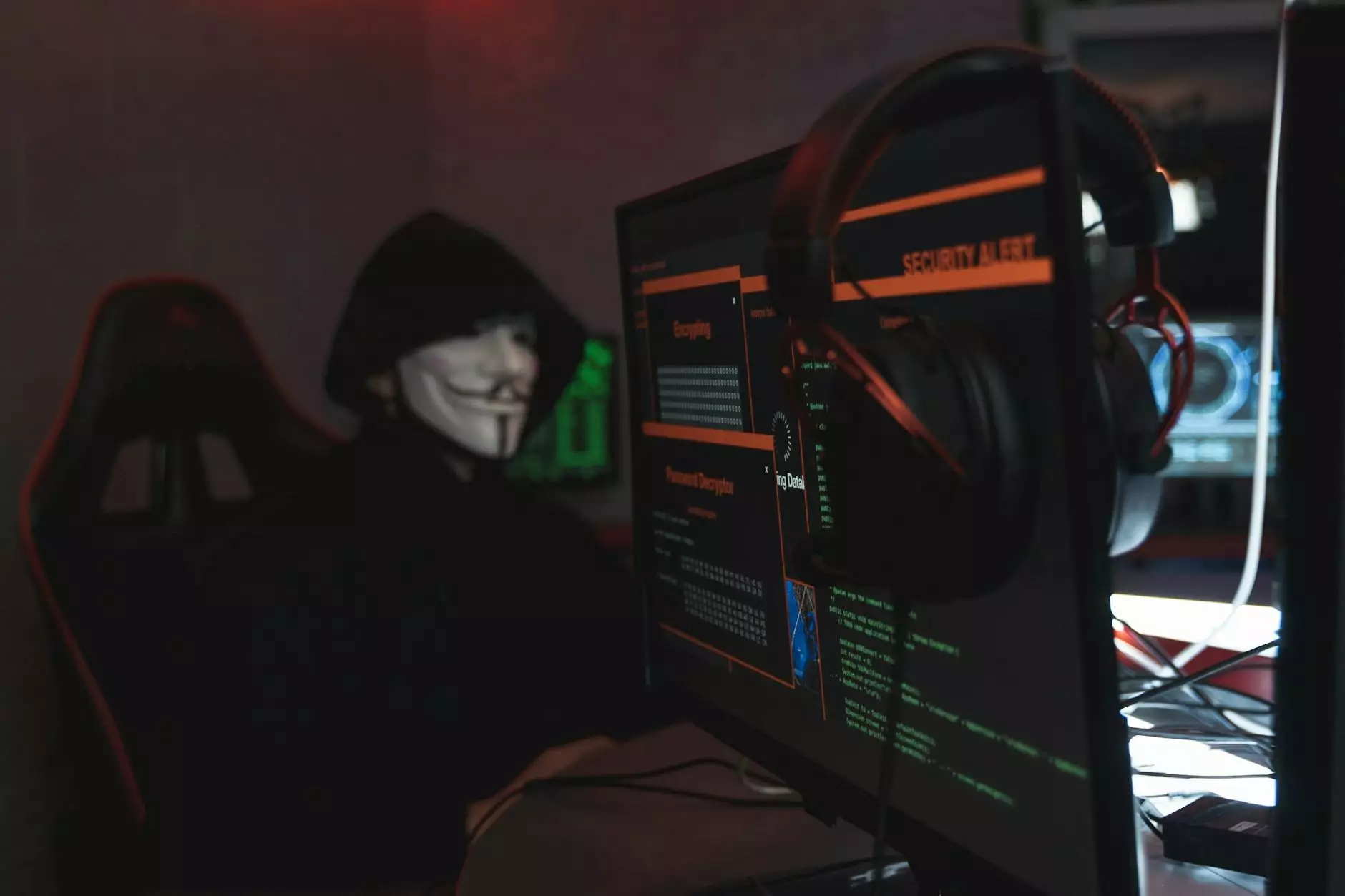
The digital age has brought *unprecedented convenience* and opportunities for businesses; however, it has also introduced a multitude of security risks. Among these risks, malware remains one of the most pervasive threats that organizations face today. As cybercriminals develop sophisticated techniques to infiltrate systems, it has become increasingly crucial for businesses to adopt advanced methodologies to protect themselves. This is where *malware machine learning* comes into play, providing innovative solutions to combat cyber threats effectively.
Understanding Malware and Its Implications for Businesses
To appreciate the value of *malware machine learning*, it is essential to understand what malware is and how it affects businesses. Malware, short for malicious software, encompasses any software deliberately designed to cause damage to a computer, server, or computer network. The various forms of malware include:
- Viruses: Programs that can replicate themselves and infect other files.
- Worms: Malware that spreads across networks without user intervention.
- Trojans: Malicious software disguised as legitimate applications.
- Spyware: Software that gathers user information without their consent.
- Ransomware: Malware that locks users out of their systems until a ransom is paid.
The ramifications of malware attacks can be catastrophic for organizations, including financial loss, data breaches, damage to reputation, and legal ramifications. With a surge in remote work and digital transactions, businesses are more vulnerable than ever, leading to an urgent need for effective security measures.
The Evolution of Security Solutions
Traditional security solutions often employ signature-based detection methods, which rely on known malware signatures to identify threats. However, this approach is increasingly outdated due to the rapid evolution of *malware machine learning*. It offers a more dynamic and intelligent means of threat detection and prevention.
Why Machine Learning Matters in Malware Detection
Machine learning, a subset of artificial intelligence, enables systems to learn from data, identify patterns, and make decisions with minimal human intervention. In the context of malware detection, machine learning can analyze vast amounts of data—far beyond human capabilities—to identify anomalies and predict potential threats. Here’s why this technology is critically important:
- Adaptive Learning: Machine learning models can continuously improve their accuracy by learning from new datasets, ensuring they remain effective against evolving malware.
- Behavioral Analysis: Rather than solely relying on signatures, machine learning algorithms can detect suspicious behavior patterns that may signal an attack.
- Reduced False Positives: Enhanced predictive capabilities lead to fewer false alarms, allowing IT teams to focus on genuine threats.
- Real-Time Protection: Machine learning can respond to threats in real-time, thereby minimizing the impact of attacks.
How Malware Machine Learning Works
The implementation of *malware machine learning* consists of several steps:
1. Data Collection
Organizations collect extensive data from various sources, including system logs, user behavior, and external threat intelligence. This dataset serves as the foundation for model training.
2. Feature Engineering
Data scientists analyze the raw data to identify which features (variables) best predict malware activity. This process is crucial for building effective models.
3. Model Training
Machine learning algorithms are trained on the pre-processed data, learning to differentiate between normal and malicious behavior. Common algorithms include:
- Decision Trees: Easy to interpret and visualize, used for classification.
- Random Forests: Ensemble technique that improves prediction accuracy by combining multiple decision trees.
- Neural Networks: Particularly effective for complex data patterns, including deep learning methods.
- Support Vector Machines (SVM): Used for classification tasks and effective in high-dimensional spaces.
4. Model Evaluation
Once trained, the models undergo rigorous evaluation using test datasets. Metrics such as precision, recall, and accuracy help determine the model's effectiveness in detecting malware.
5. Deployment
After successful evaluation, machine learning models are deployed into security systems, providing ongoing protection against emerging threats.
Real-World Applications of Malware Machine Learning
Businesses in various sectors are beginning to embrace *malware machine learning* as a viable solution to their security challenges. Here are some notable applications:
1. Financial Services
The financial sector is a prime target for cyber attacks. Machine learning models can analyze transaction patterns to identify fraudulent activities in real-time, significantly reducing the risk of financial losses.
2. Healthcare
With the increasing amount of sensitive health data stored digitally, healthcare organizations utilize machine learning to secure patient information against breaches, ensuring compliance with regulations like HIPAA.
3. E-Commerce
E-commerce platforms increasingly adopt machine learning to protect customer data and transaction information from malware and phishing attempts, fostering trust with their users.
4. Telecommunications
Telecom companies are implementing machine learning solutions to predict and mitigate distributed denial-of-service (DDoS) attacks, safeguarding their communication networks.
Integrating Malware Machine Learning into IT Services
For businesses looking to integrate *malware machine learning* into their IT services, several steps must be taken. Consider the following integration strategies:
1. Partnering with Experts
Engaging a specialized IT service provider like Spambrella can help organizations leverage advanced malware machine learning technologies tailored to their specific needs.
2. Investing in Infrastructure
A robust IT infrastructure is crucial for deploying machine learning solutions. Businesses should evaluate their current systems and make necessary upgrades to support these advanced technologies.
3. Continuous Learning and Adaptation
The cybersecurity landscape is continuously evolving; therefore, organizations must remain adaptable by regularly updating their machine learning models with new data.
4. Training Staff
Staff should undergo training to understand the functionalities of machine learning in cybersecurity. This knowledge enables them to respond effectively to alerts generated by these systems.
Challenges and Limitations
While malware machine learning presents numerous advantages, there are challenges and limitations to consider:
1. Quality of Data
The efficacy of machine learning models heavily relies on the quality and quantity of data used during training. Inadequate or biased data can lead to poor performance.
2. Complexity of Implementation
Implementing machine learning solutions can be complex, requiring expertise and careful planning to ensure that models are effectively trained and deployed.
3. Evolving Threat Landscape
Cyber threats are continually evolving, and cybercriminals often adapt quickly. This requires businesses to keep their defenses up-to-date and continuously refine their machine learning models.
Conclusion
In conclusion, the integration of *malware machine learning* into IT services and security systems presents a *holistic approach to combatting cyber threats*. As businesses navigate the complexities of the digital landscape, implementing advanced security measures powered by machine learning stands out as an essential strategy for safeguarding assets and maintaining consumer trust.
As organizations like Spambrella lead the charge in providing innovative IT services, embracing the capabilities of machine learning not only enhances security but also empowers businesses to operate more efficiently and confidently in a digitally connected world.
Investing in cutting-edge technologies is no longer a luxury but a necessity for any business aiming to thrive in the current environment. By prioritizing the adoption of *malware machine learning*, companies can stay ahead of evolving threats and ensure a resilient future.